Social media has emerged as a dynamic platform for individuals to freely express their opinions on various topics, from everyday personal experiences to major political debates. However, despite the vibrant activity on these platforms during critical events, traditional analytical models often misrepresent or only partially capture public opinion. Most models typically focus on the more vocal, active content creators while ignoring the silent yet crucial majority of users. While these individuals are less visible in digital conversations, they represent a significant part of the public’s opinion and, as part of society, will influence the outcomes of democratic processes.
Recognizing the importance of these overlooked groups, we have developed an innovative method to shed light on the stances of silent users, providing a more accurate and comprehensive picture of the social media landscape. Our approach captures the voices of frequent content creators and the more subtle but powerful influence of those who consume this content without actively engaging in the discussion. To validate our methodology, we applied it to analyzing two real-world datasets from constitutional referendums, demonstrating its effectiveness in providing a deeper, more comprehensive understanding of public discourse on social media.
The Method
Our research methodology uses a systematic, multi-step approach to analyze public stances on social media. For our case study, We collected a focused dataset from Twitter, targeting politically informed users (news outlets’ followers) and expanding over nine to ten months. This selection was designed to capture the public discourse leading to the referendums.
During the analysis phase, we automatically extract the most frequently used hashtags and manually identify those related to our topic of interest. Many of these hashtags supported or opposed the referendum process (e.g., #I_Approve or #IvoteNo), providing initial insights into active users’ attitudes. Although we do not need to annotate hashtags related to other topics, we keep them for the next steps as we leverage users’ engagement across multiple issues. Even if users are “silent” about the referendums, they may still participate in other discussions and interact with different hashtags.
We constructed interaction networks between users and hashtags (not just referendum-related but all hashtags), which constitute the basis of our predictive analysis. Our model uses these user-hashtag interactions and the user-user interactions between active and silent users to predict users’ level of interest and stance in the referendum topic by association. For this, we estimate the user’s affinity to every hashtag, even for those with which they did not explicitly interact.
To improve the accuracy of our predictions, we incorporated several layers of data into our analysis, including following, mentioning, or retweeting interactions. This enriched approach allowed us to develop a robust model that effectively predicts users’ affinities and attitudes based on their relations with other users and topics.
Our method was extensively tested, confirming its effectiveness in resolving nuanced user positions on polarized issues with a particular focus on the online silent majority. Moreover, the model allows us to better understand users’ stances by representing them in a continuous discussion space rather than discrete categories such as “in favor” vs. “against”. Users with a higher affinity toward negative referendum-related hashtags (i.e., hashtags expressing opposition to the referendum’s proposal) can be classified as “Opponents”. On the other hand, users with a high predicted affinity toward positive hashtags will be classified as “Supporters” (See Figure below). However, a finer-grain analysis is possible by looking at the distribution of the users over the space and considering if they have a softer (closer to the center) or a more rigid (closer to the extremes) stance on the topic.
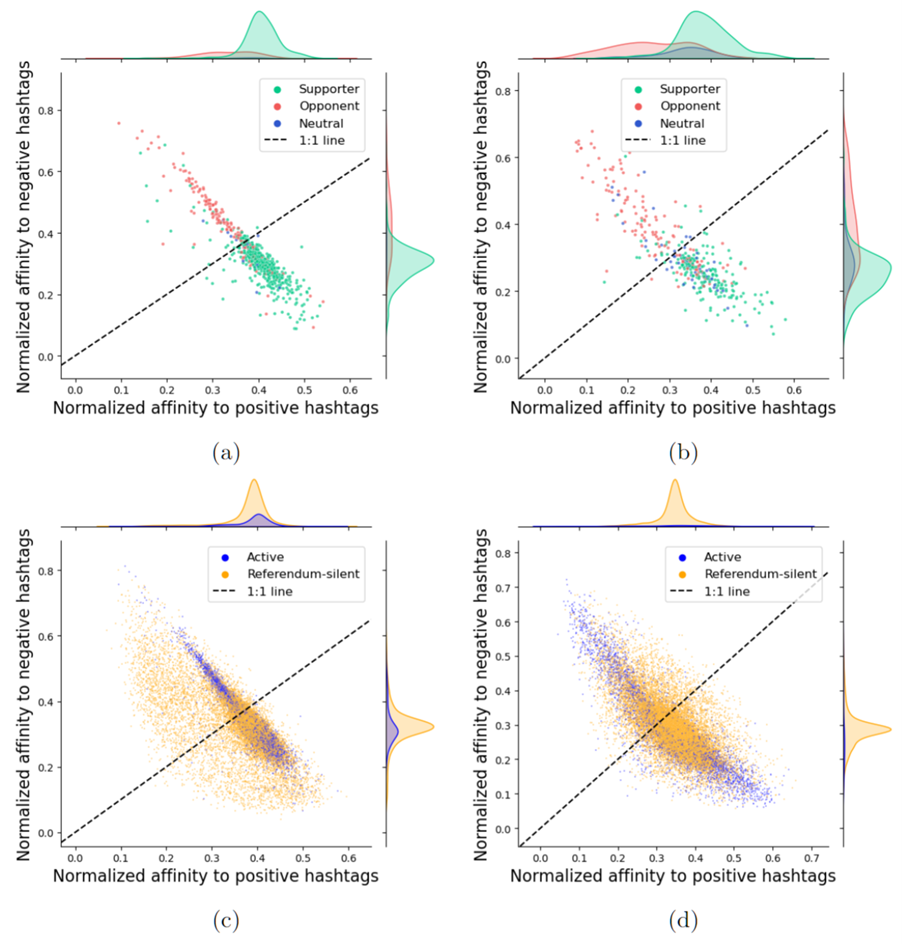
In addition, we evaluated the robustness of our model regarding the selected referendum-related hashtags. For this, we examined the impact of manual hashtag annotation on the accuracy of model predictions. We found that, at least for the studied topic, the model achieved high accuracy after including a few frequently used hashtags that were aligned with either positive or negative opinions related to the topic. Increasing the annotation effort beyond these initial subsets has only a relatively small impact on the model’s performance.
The Analysis
Beyond evaluating our model on various metrics, we also used the model predictions alongside the learned representations of users and hashtags to demonstrate prospective applications and downstream analyses.
In particular, we observed the differences between supporters and opponents of the referendums (see Figure above: (a) and (b), as well as between active users and those silent on this topic (see Figure above: (c) and (d)). For example, Figures (c) and (d) show active users’ stances are more concentrated and polarized. In contrast, referendum-silent users show a broader range of stances, suggesting a more complex discussion space for this referendum topic. These results also highlight the concern about potential bias in the analysis when relying solely on active participants.
In addition, we uncovered different patterns of the discussion tone and related interests among various groups of users. For example, from the predicted user-hashtag interactions, we see that supporters of the referendum tend to also favor other social movements and reforms and often oppose the conservative government. Conversely, opponents tend to oppose social movements, support the police, and hold more conservative views.
This ability to identify and understand different user behaviors and preferences for opposing camps showcases the analytical power of our approach, making it a valuable tool for interpreting and contextualizing complex social media landscapes.
The Conclusions
Overall, by taking the “silent majority” into account, our approach provides a more democratic and accurate reflection of societal opinion, especially during contentious events. This method provides an example of how CRiTERIA uses advanced AI models to gain insights into complex interactions within social media networks.
However, future research should address some identified challenges. For example, hashtag hijacking and demographic biases on platforms like Twitter may hinder the representativeness and generalizability of our results to the broader offline population. Although including less active users is a step in the right direction to reduce these biases, analysts must remain cautious and evaluate the results’ applicability on a case-by-case basis.
More results and in-depth analysis can be found in the paper “Migration reframed? A multilingual analysis on the stance shift in Europe during the Ukrainian crisis” now available for download in the CRiTERIA’s publication hub.
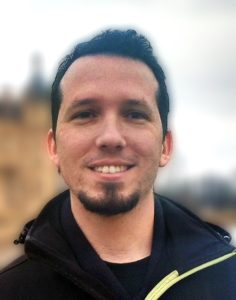
Erick Elejalde, PhD
Erick Elejalde received the M.Sc. and Ph.D. degrees in computer science from the University of Concepción, Concepción, Chile, in 2013 and 2018, respectively. He is currently a Post-Doctoral Researcher with the L3S Research Center, Leibniz University Hannover, Hannover, Germany. His research interests include computational social science, online media-behavior modeling, and social networks.

Zhiwei Zhou
Zhiwei Zhou is a research assistant and Ph.D. student at L3S Research Center. He completed his master's degree in Computer Engineering from Leibniz University Hannover. His research interests include social network analysis, stance detection, and graph neural networks.
Banner image by Gerd Altmann on Pixabay.